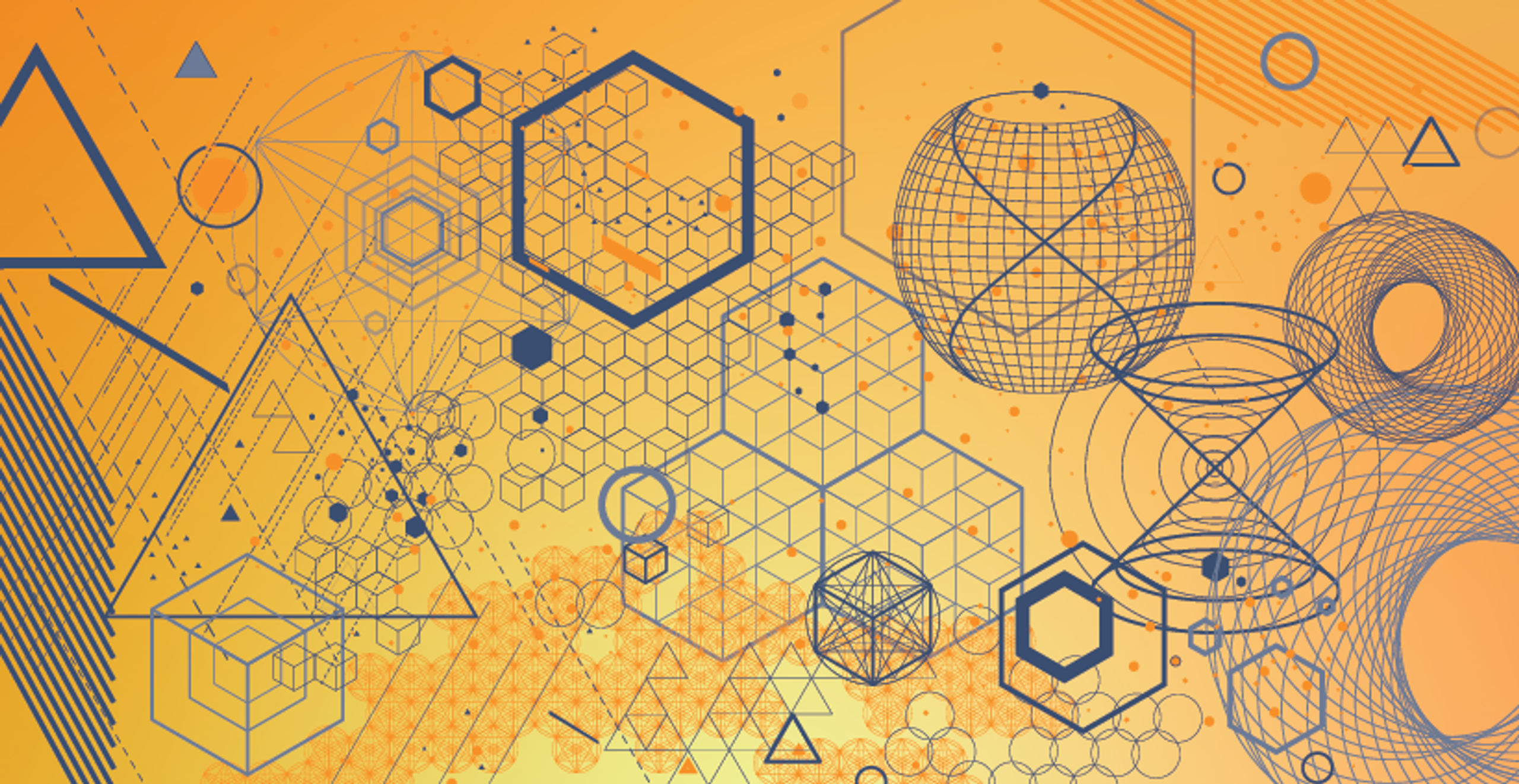
Characteristics of a Well-Designed Experiment
January 31, 2024In the age of big data, it is tempting to throw out traditional statistical ('small data') methods of inference, such as design of experiments, in favor of the new supervised and unsupervised approaches for large and unstructured datasets, which take the large volumes of data available and crunch it into some semblance of insights.
However, keep in mind that these methods simply look for patterns observed among the included variables – they do not attempt to explain the nature of those associations or, indeed, to discern between coincidental associations and those arising from a true underlying causal mechanism. While there is undoubtedly a case to be made for big data methods depending on the nature of the problem, the available resources, and the specific goals of the analysis, controlled experiments are still the gold standard when it comes to proving causation.
A Strategic Approach to Experimental Design
In our DOE webinars last month, we made the case for using simple but rigorous experimental designs to establish cause-and-effect relationships between multiple factors and the desired output. While keeping things simple is always the recommended approach, there are plenty of cases where the nature of the underlying process is complex, which in turn necessitates a more nuanced approach to modeling. In such cases, your experimentation strategy might be iterative, learning and fine-tuning the questions at each step to gain greater understanding. Your journey may look like this:
Step 1: Use a screening design, which can be of relatively low resolution, to sift through the large number of potential factors identified through brainstorming and root cause analyses with a minimal number of runs.
Step 2: If the design finds some of the factors and/or their interactions to be significant with respect to the response variable, focus on this subset of factors in the next step with a higher resolution fractional or full factorial design, with replicates, that will not only confirm the significance of the effects but also allow you to find the optimal settings of the factors to achieve the desired goal for the response. You can also add center points to the design at this stage to confirm or rule out curvature in the response space.
Step 3: If the previous analysis gets you close to the target response but not spot on, create a new design by adding previously overlooked but potentially significant factors and/or by tweaking the levels of the existing factors in the direction of the desired effect. This will move or expand the feasible region for the optimal response. Suppose curvature is present in the response space. In that case, you can augment the design with 'axial' points (factor settings at a specified distance from the center of the design) to convert the design into a response surface methods (RSM) design, allowing you to estimate and model the curvature, resulting in a predictive model.
Note: Because an RSM design is used to refine the model and make reasonable predictions rather than determine whether an effect is real, the precision of its predictions (or, conversely, prediction error) is a more important consideration than power at this stage.
Step 4: Let's say the final model yields the operating conditions that result in the optimal response value/s. Is your work done? Not so fast! Without confirming your results in the production environment, your results are simply conjecture. Piloting your results will help validate the success of your experiment, demonstrate the impact of the proposed changes, and verify that these changes did not unintentionally break another part of the process.
Best Practices for Effective Experimental Design
Here are some things that pay to keep in mind and address early on to avoid potential issues late in the analytical process:
- Get a good understanding of the process or system you will experiment on, or involve a process expert early on. Inadequate knowledge about the system can lead to selecting irrelevant factors or failing to identify critical variables driving the system. You may find out, for instance, that the response space is non-linear in some of its relationships – knowing this, you can include center points in the design to verify the curvature or, if already verified, run a response surface model to specify the correct model.
- Choose a design that is 'up to the task' for the purpose. If you suspect interactions among factors are driving the process response, do not opt for the lowest resolution (res III) design – keep in mind that such a design will make it impossible to distinguish between significant main effects and aliased (confounded) two-factor interaction effects.
- Power the design to detect (find significant) a meaningfully important (in the practical, 'real world' sense) effect size. Many DOEs have failed to identify significant effects (even if they do exist) for want of sufficient power. The smallest effect size anticipated, the chosen level of significance, number of replicates, and experimental error/variability are the levels available to you to improve the power of your design.
- Map out the process to identify potential nuisance factors in the system and control them at a set level or account for them in the design as blocking factors to avoid any untoward result, such as the factor masking a real effect.
- An easy way to increase the statistical power of your design is by taking repeated measurements at each design run. This reduces the measurement error (variability), thereby improving the estimates' precision and, ultimately, the design's power.
Choosing the Right DOE Software
When planning a DOE, it is essential to use statistical software that helps you design and analyze the most appropriate experiment to answer your research questions. EngineRoom's DOE tool has built-in features specifically designed to guide you through selecting a design streamlined in favor of the resources needed but also powerful enough to detect an effect if it exists.
It provides a comprehensive list of full, fractional, and general factorial designs to cover a wide variety of DOE scenarios. It also allows you to run automated algorithms to select the best model for the data, making it easier to draw conclusions and take informed actions. Using EngineRoom for your designed experiments can save time, reduce costly errors, and help make data-driven decisions.
Alternative Techniques to Design of Experiments
If you're faced with a situation that makes it difficult to run a regular DOE, a different design or procedure might be available to continue the study. Some examples:
- An experiment with a non-continuous (say, binary or nominal) response variable can be modeled using a logistic or other appropriate non-linear regression method. The fact that the analysis is based not on observational but experimental data allows you to make causal inferences.
- Suppose the design includes factors that are very expensive or difficult to vary over different levels. In that case, you can employ a split-plot design that assigns a tiered randomization scheme – one for the hard-to-change factor(s) and the other for the remaining factors.
- You may have measured certain environmental variables (say, ambient temperature or humidity) or process variables you could not control during the experiment. These can be included in the analysis as covariates, thus accounting for their effect and reducing experimental error.
- When the process under study is in the design stage, i.e., no actual process exists to execute the experiment on; you can use process modeling and simulation techniques to model the results of the experimental runs.
The successful execution and implementation of a design of experiments study requires a combination of process knowledge, careful planning, and effective communication with stakeholders. Remember, the key is to be flexible and adopt an attitude of continuous learning and adaptation throughout the experimental process.
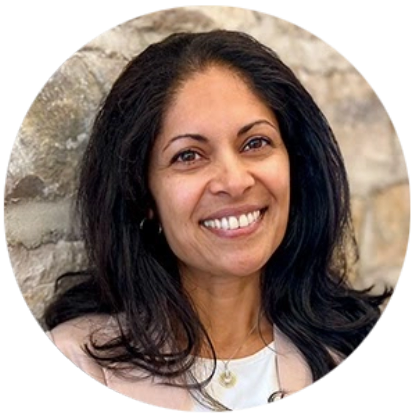
Principal Statistician • MoreSteam
Smita Skrivanek was MoreSteam's first full-time employee on the payroll. Currently, she leads research and development for EngineRoom, including its patented hypothesis testing and DOE wizard elements. Throughout her tenure, she has been responsible for building curriculum, coaching, reviewing projects, and assisting students with their advanced statistical questions. Smita previously taught college-level Statistics courses.
Smita has a Masters in Statistics from The Ohio State University and an MBA from Indiana University Kelley School of Business.