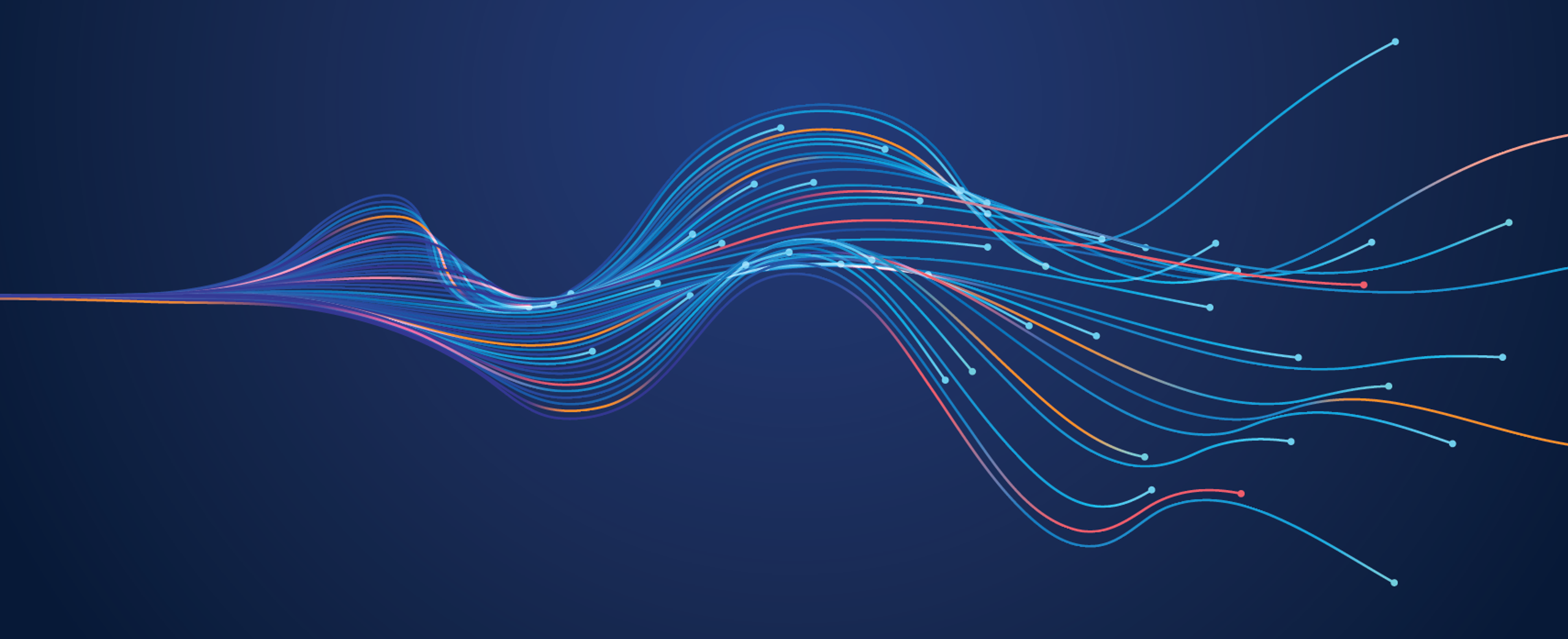
The Next Essential Tool for Root Cause Analysis
December 12, 2024Root cause analysis (RCA) is the detective work of process improvement—the methodical search for the hidden reasons behind a problem. Traditionally, tools like fishbone diagrams or process flow maps have helped us piece together the "why" behind issues. These tools are certainly useful but can sometimes fall short for today’s challenges. Why? Because the problems we face are no longer simple.
Modern organizations generate mountains of data. Uncovering answers within these data troves is no simple task. The search is often complicated by overwhelming noise that must be sifted through, true factors that can be obscured by others due to multicollinearity, or intricate interactions and dependencies between factors that are challenging to untangle. It’s like trying to find a needle in not just one haystack but a whole barn of haystacks.
That’s where machine learning proves useful—not as a replacement for traditional RCA tools, but as a way to enhance that toolkit. By processing large datasets quickly and identifying patterns that might be hard to spot manually, machine learning helps streamline and deepen the search for intricate, complex, or hidden root causes. And it doesn’t stop there. It equips teams to predict problems before they emerge, to better notice near misses and adjust before a failure occurs.
The Power of Machine Learning in RCA
Machine learning brings a data-driven precision to RCA that wasn’t previously possible. Here’s how it amplifies our problem-solving capabilities:
1. Enhanced Data Analysis
With the ability to process vast amounts of data, machine learning reveals patterns, correlations, and anomalies faster than traditional methods. For industries drowning in data—like manufacturing, healthcare, and finance—this speed is invaluable.
2. Predictive Insights
Rather than merely identifying what went wrong, machine learning anticipates future issues by spotting trends and signals. This predictive capability allows teams to shift from reactive firefighting to proactive prevention.
3. Real-Time Monitoring
Machine learning models continuously analyze systems, flagging anomalies as they happen. This real-time capability ensures problems are addressed before they escalate which can be a game-changer for industries in which every second counts.
4. Uncovering Hidden Complexity
When a problem stems from a tangled web of interrelated factors, traditional methods often fall short. Machine learning thrives here, particularly with techniques like classification and regression trees (C/RT). These tools reveal nuanced relationships, helping teams pinpoint root causes even in the most complex scenarios.
Bringing the Concept to Life: A Case Study
Consider a national bank grappling with an alarming rise in customer churn. Churn is notoriously complex—customers rarely leave for a single reason. Instead, the causes are often buried in a mix of behaviors, preferences, and external factors. Traditional RCA tools might identify general patterns, such as customers leaving after changes in service fees, but they struggle to go deeper.
To address this, the bank turns to machine learning, using a decision tree to analyze a rich dataset that includes variables like age, education, income, tenure, and account activity. The results are revealing:
- Segment Discovery: The C/RT identifies a specific segment of customers—long-tenured, high-income individuals with low account activity—as the group most likely to churn.
- Contributing Factors: Further analysis shows these customers feel undervalued compared to newer, high-income clients who are offered attractive onboarding perks.
- Predictive Power: The model not only explains past churn but predicts future at-risk customers with a high degree of accuracy.
Armed with these insights, the bank can take targeted action:
- Personalized Retention Strategies: They design loyalty programs specifically for long-tenured customers, offering rewards and incentives that acknowledge their value.
- Proactive Outreach: Customer service teams are equipped with predictive churn lists, allowing them to contact at-risk clients before dissatisfaction turns into departure.
- Enhanced Onboarding Fairness: The bank adjusts its onboarding perks to include upgrades for loyal, existing customers, ensuring they feel as valued as new clients.
These actions lead to measurable results: a significant reduction in churn, increased customer satisfaction scores, and a more equitable perception of the bank’s value proposition. This is the power of machine learning in RCA—it not only diagnoses the issue but provides a roadmap for resolution.
Navigating Challenges in Machine Learning-Driven RCA
As with any advanced tool, there are hurdles to consider when integrating machine learning into RCA:
- Data Quality: A machine learning algorithm is only as good as the data it’s fed. Poor-quality inputs lead to unreliable outputs. If your data contains bias or preconceptions, you might miss important insights or overlook critical variables, skewing the results and potentially reinforcing systemic issues.
- Expertise: Successful implementation requires some degree of specialized knowledge in both data science and RCA. Building this expertise—or partnering with those who have it—is key.
- Ethical Considerations: Handling sensitive data comes with a responsibility to protect privacy and ensure ethical use.
- Bias: Machine learning models can inadvertently reflect and reinforce biases present in the training data. This can lead to unfair or inaccurate conclusions, especially in datasets that lack diversity or represent historical inequalities.
The Evolving Role of RCA in Process Improvement
The goals of RCA have always been the same: discover, correct, and prevent. But the complexity of today’s challenges demands new tools and approaches be added to our toolkit. Machine learning not only sharpens our ability to identify root causes but also enables us to anticipate and mitigate issues before they arise.
By blending the strengths of traditional methods with the advanced capabilities of machine learning, organizations can move from reactive problem-solving to proactive, data-driven improvement. It’s an exciting shift—one that offers both challenges and opportunities.
Excited to learn more? Join us for our Advanced Root Cause Analysis for Quality and Process Experts Master Series to build your expertise in advanced root cause analysis.
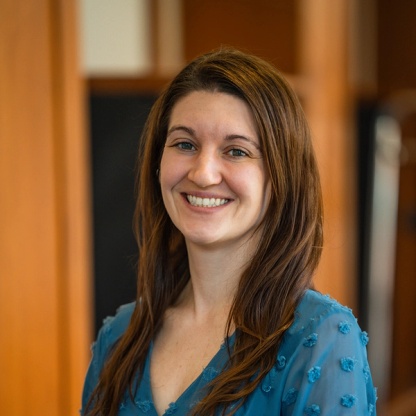
Vice President of Marketing • MoreSteam.com LLC
Lindsay Van Dyne joined the MoreSteam team in 2014. She is responsible for developing and executing the company's marketing strategy. Her marketing experience includes technical aspects of search engine optimization (SEO), digital content marketing strategies, lead generation, website development, event management, and partner relationships. Before switching to the marketing team, Lindsay spent several years as MoreSteam's eLearning product manager. During that time, she led the eLearning team through an entire UI transformation, developing a new user interface for training, expanding the language offerings significantly, and adding features like notes & highlighting and a user dashboard for training stats. Lindsay's drive and spicy personality bring a fresh perspective to the leadership and marketing teams, encouraging others to think creatively.
Lindsay received a B.S. in Chemical Engineering from the University of Notre Dame and a B.S. in Computational Physics and Mathematics from Bethel College.