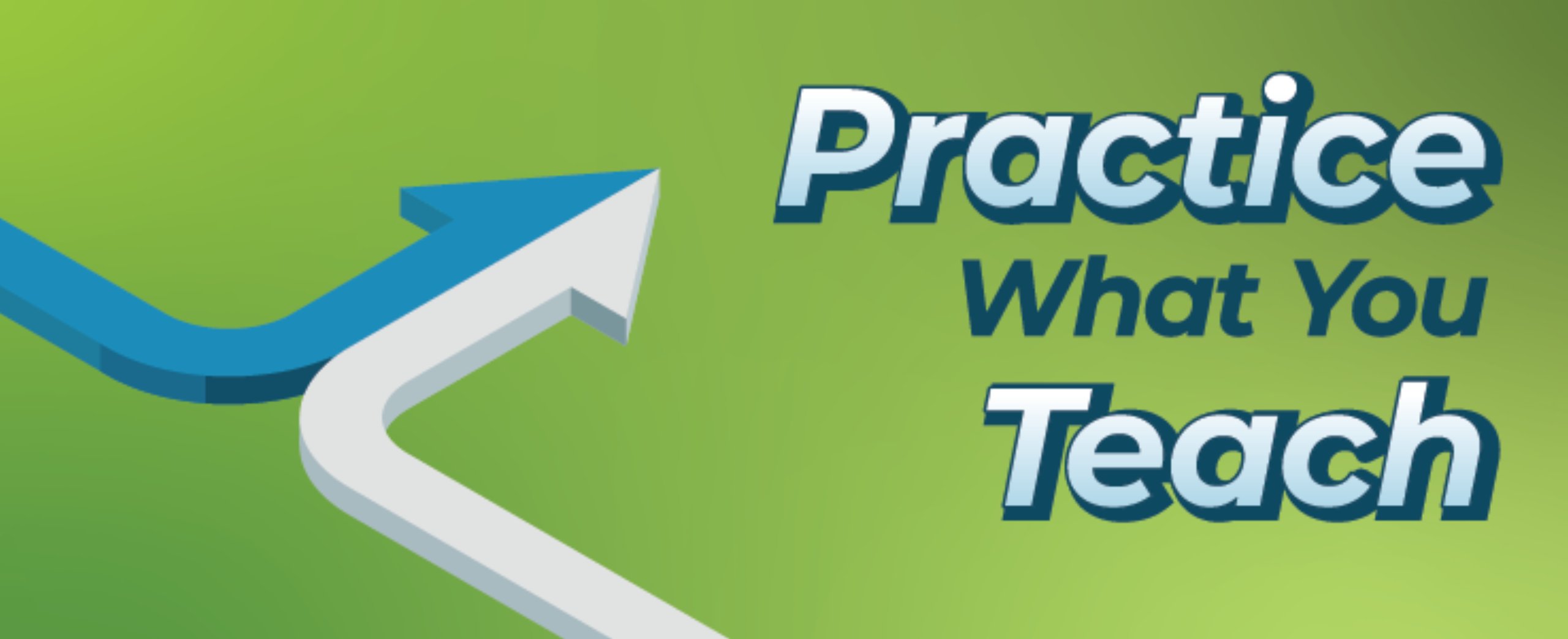
Applying Lean to Six Sigma Training
September 14, 2022Fellow Master Black Belt and Lean Six Sigma practitioner, Kevin Keller, shares his thoughts on why Lean Six Sigma processes are difficult to see to fruition.
I have been teaching and applying Six Sigma since 1989. Although my initial start was directly tied to the manufacture of products, I soon began utilizing those tools and techniques in every function of the companies I worked for. Today, most of the LSS work I do is supporting non-manufacturing operations.
During the early part of my career, Lean methodology was a separate initiative for several major reasons. Although the problem-solving journey was similar, the tools and techniques were quite different, and the need for heavy statistics was not there. Along the way, people recognized the opportunity of merging the two. Both approaches follow the scientific method, whether the roadmap is PDCA or DMAIC, and many tools are shared.
Yet, what we didn't do is lean out Lean Six Sigma when combining the two. As Master Black Belts (MBBs), we often scratch our heads wondering why LSS projects launched don't get completed. In some respects, LSS can be anti-Lean unless we're strategic about how we go about teaching and implementing LSS.
Figuring out the Correct Tools to Solve the Problem
Back in 1984, I first learned PDCA problem-solving through the Juran Institute's training. All engineers at Texas Instruments had to go through "Juran training." In our group, three teams of engineers were tasked with doing a project to support our training. After the training, my team was the only team that solved the problem assigned.
We were first to present, and at the end of the presentation, the plant manager forever doomed further implementation by saying, "What's missing from this presentation?"
The air was sucked out of the room.
One voice piped up, "There's no histogram of the data."
"Right. What else?" This feeding frenzy went on for a few minutes, identifying every tool we didn't use or present.
Never mind that Juran specifically stated, "Only use the tools you need to solve the problem." And, oh, by the way, plotting a histogram would have been inappropriate on that project.
As MBBs in class, we preach, "use the correct tools to solve the problem." Yet as MBBs, we often require tools to be completed regardless of whether they add value or make sense.
Lean Six Sigma methodology tools were predominantly developed around making widgets. All the Lean and/or Six Sigma tools help us make devices rapidly, cheaply, and consistently.
Many of these tools can be used for transactional and other projects. But not all, and as MBBs, our job is to make sure the project leader (aka belt) answers the critical questions and does not use specific tools that might not apply.
Stay up-to-date on all things MoreSteam by following us on LinkedIn
Diving Deeper Into a Few Classic Examples
There's no process capability or yield/sigma calculation.
The project's goal was to reduce the process average for a support process. There was no upper specification limit. Every capability statistic in LSS requires a specification limit (and I've seen belts use the average as the specification! - but that's another story).
Calculating by using the average or making up a nonsense specification just to check a box is anti-lean. What is value-added is to assess the behavior of the process average over time. The assessment of "what is my baseline average?" is critical to the project and will be used at the end for comparison. Calculating how many values fall in the specification when there is no specification is a non-value activity.
There's no Measurement System Analysis.
True, but there was an investigation into the reliability of the data. When we measure widgets, MSAs are important; critical if those measurement devices are used for making go/no-go decisions about a product meeting customer expectations. We do an MSA to answer the question, " Are our data reliable?"
Answering that question is the goal, not to answer the question, "What did the MSA say?" If the data and collection lend themselves to an MSA, great.
What matters most is answering, "Can you trust the data to make decisions?" That every belt should be able to discuss and defend. Many non-manufacturing LSS projects don't support an MSA being conducted.
"I have to transform the data because the A-D statistic p-value is < 0.05". Usually anti-lean. It may be appropriate if we are putting a product on a truck and shipping it to a customer.
We need to accurately understand what is in the truck, and a transformation might help. But what about other assumptions required?
- "The process is stable over time." If we're working on a belt project, we may find the assumption of stability untrue if the process has worsened over time.
- "The data come from a single population." This assumption is often (almost always) not true.
If either of these assumptions are false (usually the case with belt projects), then the statistic is flawed anyway; why slow the project down by performing an incorrect calculation? If we actually did a good job in the "Relevant" portion of the SMART objective, then the project is strategically important to the company. Data transformation might give a better estimate of already poor performance, but for what value?
The directors/VPs who assigned the project can see the bleeding dashboard or budget. They don't need a precise estimate of lousy performance. The project will make the actual process metric better.
Is statistical rigor non-value-added here? Teaching the transformation technique might be value-added if the belt lives on the manufacturing floor in their actual job and deals with non-normal data for a living. It is typically non-value-added for everyone else.
As a practitioner, I have pushed all data analyses beyond assessing baseline performance and data validity to the Analyze phase. Asking the question, "Why do we have a performance gap?" allows the answers to select what tools to use next.
That is a Lean approach to LSS vs. conducting all stratification in the Measure phase, which might not be needed or used. The Lean methodology allows the questions and answers to drive the diagnostic journey and use the proper tools when required.
Identifying the Problem
For two clients, I was asked to study why they don't complete LSS projects that they launch. In both cases, the results were identical. Those that took a long time in the Define or Measure phase had a lower probability of completion than those that went through those phases quickly.
In talking with the belts, there is palpable excitement about studying the data and digging into solving the problem. No one enjoys the Define and Measure phase. Fundamental questions must be answered to keep aligned with the business, but don't slow the process down by insisting upon "rigor" or "checking the box" to ensure full use of the Body of Knowledge. Do what is value-added and no more - that is the essence of Lean methodology.
The problem-solving journey is sound. The tools used along the way are just that; tools, not an end. We should all eat our own cooking and be more lean in our approach to teaching and applying Lean Six Sigma!
For more think-pieces like this one, subscribe to our newsletter!
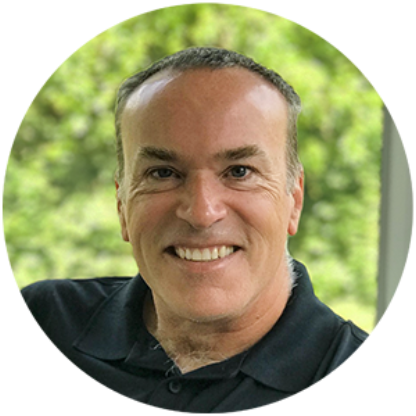
Master Black Belt • MoreSteam Client Services
Kevin Keller is a professional statistician and quality professional with over 30 years of experience teaching, coaching, and leading Lean Six Sigma project teams from the shop floor to the enterprise levels. He began his career as a process engineer at Texas Instruments and then served in a Master Black Belt role at MEMC Electronic Materials for 15 years. Kevin advanced to manage quality systems for AB‐InBev and eventually accepted a Master Black Belt position for AB‐InBev's North American Zone. Kevin earned a BS in Chemical Engineering from Missouri University of Science and Technology and a Masters in Applied Statistics from The Ohio State University. He also is a certified Lean Six Sigma MBB.