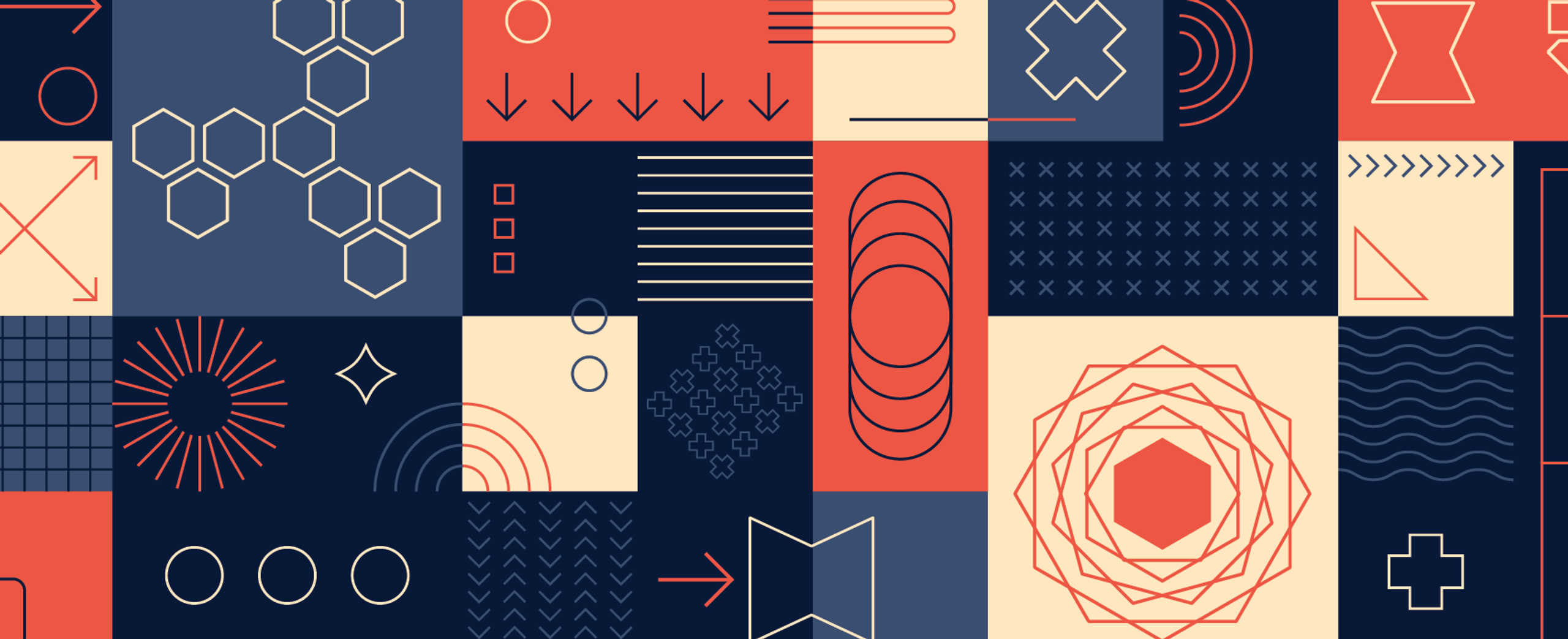
5 Common Process Modeling Mistakes and How to Avoid Them
March 27, 2025If you’re still on the fence about whether process modeling is the next skill you need to develop as a continuous improvement practitioner, check out our other blogs on why discrete event simulation (DES) is a game-changer for Lean transformations. But if you’re already convinced and ready to start building models, there’s something important you should know—garbage in, garbage out.
Process modeling can drive serious results—if it’s built on the right foundation. Missteps in model design can lead to misleading outputs, unnecessary complexity, or even the false confidence that your changes will work when they won’t. As you dive in, let’s look at some of the most common mistakes people make when building their first process models—and, more importantly, how to avoid them by following a few key process modeling best practices.
New to process modeling? This overview from IBM offers a helpful introduction.
1. Improper Scoping
One of the biggest pitfalls in process modeling is failing to define an appropriate scope. Many modelers either go too broad, trying to capture every upstream and downstream element of the process, or too narrow, obsessing over minute details that don’t impact the overall system behavior. Before building a model, clearly define the goal. What decision are you trying to make? What level of detail is necessary to achieve that? Often, a simpler model that captures the core dynamics of the process is sufficient to analyze and test improvements.
For example, if you're modeling grocery store checkout in order to estimate staffing needs, you don’t necessarily need to model every granular step like loading items onto the belt, scanning, bagging, and reloading the cart as separate activities. A single average input-to-output cycle time for “checkout” might be sufficient for your purpose. If you later discover that one of those steps is the true bottleneck or varies significantly, you can add that detail in a future iteration of the model. Start with a level of detail that matches your decision-making needs.
It’s tempting to include everything because upstream and downstream interactions may seem relevant. However, a bloated model becomes difficult to analyze and introduces unnecessary complexity that slows you down. On the other hand, hyper-focusing on every single process step, minor variation, or exception can result in a model that is difficult to maintain and interpret. Not every detail adds value to decision-making. It’s best to start simple and add details as they are needed when validating the model against what you observe in reality.
Also, if you're dealing with an extremely large system, remember you can break it into smaller, manageable sub-models. You can use the output from one model—captured as a distribution—to drive the input demand of another. This modular approach allows for more focused analysis without losing the connection between system components.
2. Failing to Validate the Model with Real Data
A process model is only as good as the data it’s based on. If your model’s inputs don’t match reality, your outputs will be unreliable. This is a classic case of "garbage in, garbage out." Sometimes, teams build models based on theoretical assumptions rather than actual data from the process. If task durations, arrival rates, or probabilities are off, the model won’t reflect reality. Make sure the data you have regarding demand, cycle times, and other important process metrics are reliable. Even if a model is carefully built, it’s essential to test it by comparing outputs to observed process performance. Does the model behave as expected? Are the bottlenecks, queues, or resource constraints showing up where they do in reality? Run it several times and iterate if necessary.
Ensure that all inputs—cycle times, arrival rates, processing times, etc.—are grounded in real-world data. Once the model is built, run test simulations and ask, Does this reflect what we observe in practice? If not, refine the inputs until the model aligns with reality.
3. Overlooking Resource Constraints
Many models assume that resources (people, machines, materials) are always available when needed. However, real-world processes often face bottlenecks due to limited capacity. Models that don’t factor in equipment downtime, shift schedules, or staffing limitations can produce inaccurate results. If you are properly validating your digital twin with what you observe, you should definitely catch this. If a model doesn’t account for resource constraints, it may suggest improvements that aren’t feasible in practice. Ensure the model includes realistic resource constraints, such as machine utilization, operator availability, and maintenance schedules. Use queueing models to analyze potential bottlenecks.
Consider a call center with two representatives—one junior and one senior. Calls come in and are initially handled by the junior rep, but certain issues require escalation to the senior rep. If your model assumes that the senior rep is always available to take escalations, it may significantly underestimate wait times. In reality, the senior rep may already be busy with another escalated issue. If your model doesn’t account for that limited availability, it will miss a critical constraint in the system. Properly modeling the dependency between reps—and the delays introduced when escalation isn't immediately possible—is essential for accurate planning and staffing analysis.
4. Capacity Changes Based on Process Mix
Some systems have flexible capacity, depending on the mix of items flowing through the process. If a model assumes fixed capacity regardless of mix, it can distort throughput and efficiency projections.
In a hospital emergency department, a treatment room might serve multiple types of patients—those needing basic evaluation, complex diagnostic imaging, or even emergency procedures. The number of patients a room can handle per hour changes drastically based on that mix. For instance, if you’re modeling based on an average time per patient, but the patient mix shifts toward higher-acuity cases, your model will underestimate delays and required staffing. A room that typically sees four patients an hour may only see two when the cases are more complex. If your model doesn't account for the impact of patient type on capacity, it will miss important implications for throughput and patient wait times.
Understand how different combinations of items affect the shared resources in your process. Gather real-world data on how capacity shifts with varying inputs and reflect those patterns in your model logic. When possible, use condition-based logic or decision rules to dynamically allocate capacity. Run scenario testing to see how different mixes impact throughput and delay. And if capacity rules are complex or non-linear, consider interviewing frontline staff or observing the process firsthand to better capture those variations.
This kind of modeling challenge is common in healthcare, where variability in patient needs can dramatically affect throughput. We recently shared a real-world example in our webinar on reducing emergency department turnaround times, where process modeling played a key role in identifying delays and improving staffing decisions.
5. Failing to Engage Process Owners
A process model should not be built in isolation. If process owners—those who work within or depend on the process—aren’t involved, the model may miss critical insights. Just as important as “Going to Gemba,” talking to process owners, operators, and decision-makers can help you understand key process nuances that may be useful when constructing your process model. Also, lean on these same stakeholders when you review and validate the model to ensure it reflects how work is truly performed.
Involving process owners early also builds buy-in for the modeling effort. When the people closest to the work feel heard and see their input reflected in the model, they’re more likely to trust the outputs and support changes based on the simulation. Their knowledge can also help you uncover hidden constraints, variation sources, or real-world workarounds that wouldn’t show up in documentation alone.
Final Thoughts
Process modeling is an invaluable tool when done correctly. By properly scoping your model, validating it with real data, accounting for variability, considering resource constraints, and involving stakeholders, you can avoid common mistakes and apply proven process modeling best practices that lead to meaningful improvements. Whether you’re using process modeling for Lean Six Sigma, operations management, or continuous improvement, keeping these principles in mind will help you build more effective and actionable models.
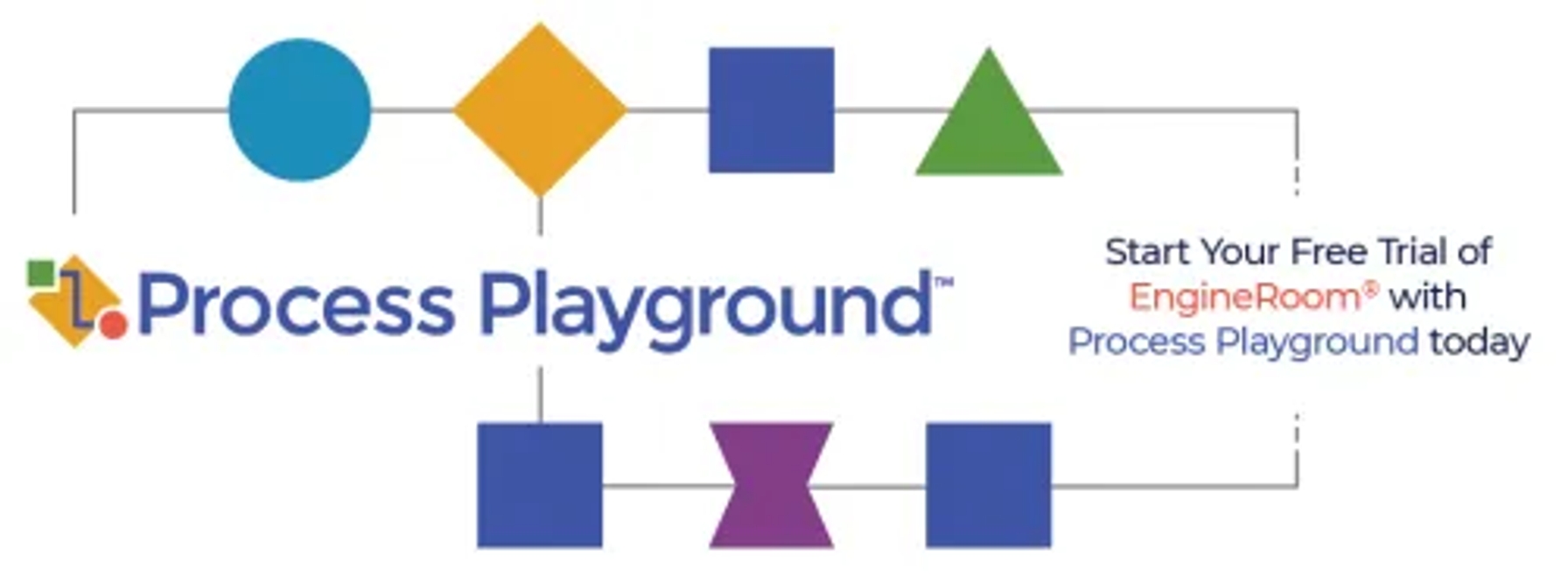
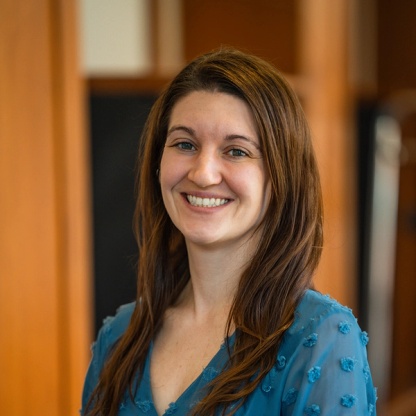
Vice President of Marketing • MoreSteam.com LLC
Lindsay Van Dyne joined the MoreSteam team in 2014. She is responsible for developing and executing the company's marketing strategy. Her marketing experience includes technical aspects of search engine optimization (SEO), digital content marketing strategies, lead generation, website development, event management, and partner relationships. Before switching to the marketing team, Lindsay spent several years as MoreSteam's eLearning product manager. During that time, she led the eLearning team through an entire UI transformation, developing a new user interface for training, expanding the language offerings significantly, and adding features like notes & highlighting and a user dashboard for training stats. Lindsay's drive and spicy personality bring a fresh perspective to the leadership and marketing teams, encouraging others to think creatively.
Lindsay received a B.S. in Chemical Engineering from the University of Notre Dame and a B.S. in Computational Physics and Mathematics from Bethel College.